At the heart of health engagement is the desire to understand people as individuals. To get to the point of true personalization with hundreds of thousands of people—or in some cases, millions—healthcare needs to leverage tools to help us predict what will resonate to drive action. This is where machine learning and predictive modeling enter the equation. But how does data bias create blind spots and effectively derail engagement?
To begin understanding data bias it’s important to remember that bias doesn’t come from machine learning algorithms, it comes from people.
Data bias happens when your data sample isn’t representative of your population of interest. It means that you may have results, but it’s not telling you the whole story so you end up generalizing and in some cases, this misinterpretation of data leaves out an entire population.
The “why” behind your data matters just as much as the data itself and the phrase “garbage in, garbage out” applies here. If you are taking your data at face value and not asking deeper questions, you are undoubtedly leaving groups of people behind because you aren’t aware of the complex layers within your data.
Here are 3 tips to consider to reduce data bias in your health engagement strategy to engage your entire population, not just the majority.
1—Be Thoughtful About Your Data Strategy
It’s essentially inescapable—all data is biased in some way or another. But if you have a data strategy that includes diverse datasets based on real examples, you’re one step closer to eliminating as much bias as possible.
A recent article by Health Data Management (HDM) said it best:
“Harmful stereotypes and inequalities influencing healthcare can create potentially damaging biases in the data on which artificial intelligence and machine learning algorithms are trained.”
HDM points to a specific example explaining that studies have shown statistically significant differences in which women are under-treated compared with men—such as heart disease—even when they have come to physicians with the same set of symptoms.
From a health engagement perspective, we’re always working to eliminate bias from our own health action program design. Bias needs to be considered from every angle so we aren’t outreaching to entire groups of people that aren’t likely at risk for certain health outcomes.
We can battle these issues with data bias by leaving out certain parameters that often create bias and finding the right way to outreach to different people. By taking into account a myriad of things and understanding real differences in language, ethnicity, socioeconomic status, and gender we can reach the right people more effectively and improve outcomes.
“We are cognizant of the variables we use to make our predictions—we aren’t using the weather to predict if someone is going is going to get a flu shot—we go significantly deeper by asking how close are they to a pharmacy? What social determinant barriers exist?”
“We need to use a broad spectrum of statistics to see people as true individuals rather than “you are in bucket 12” to get to real personalization without bias.”
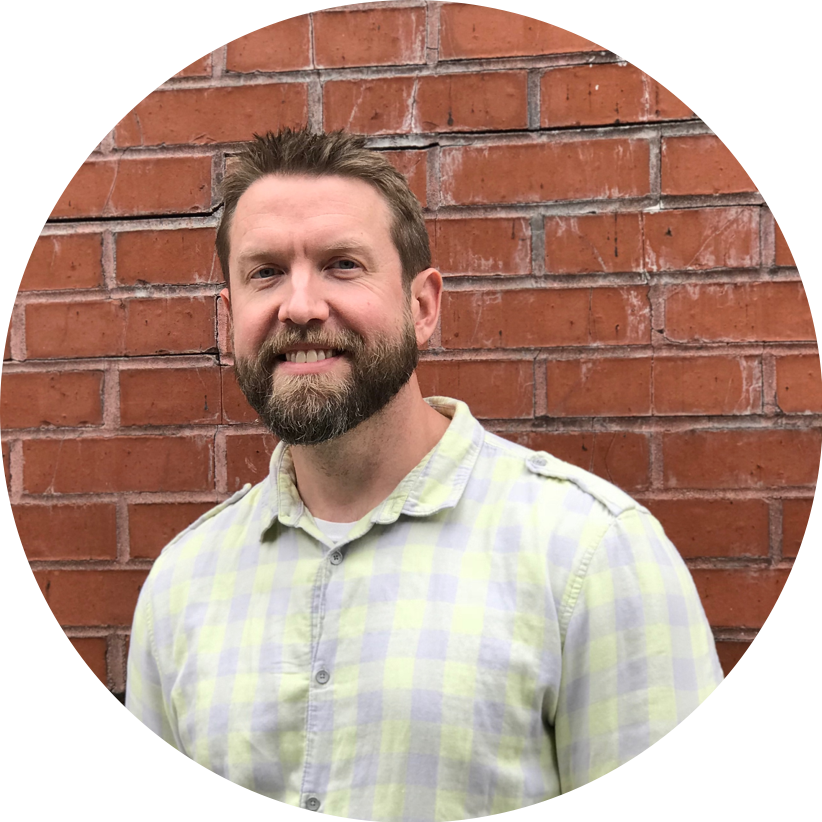
Andrew Larsen
Principal Data Scientist2—Behavioral Research is the Ying to Data Science’s Yang
The data that comes from machine learning has become a game changer for a lot of industries, including healthcare. But in health engagement, data doesn’t tell the whole story. Earlier this year we interviewed Matt Swanson, a human-centered design expert, and he filled us in on the complexity of behavioral research and the way people think. Matt said:
“Facts and evidence aren’t personal—they don’t have any concrete meaning for an individual. Facts don’t convince people, so it becomes impossible to fact people into action.”
“They may work for some people, but different things motivate different people and it’s my job to understand what those differences are so we can help health plans and providers engage their members and patients more effectively.”
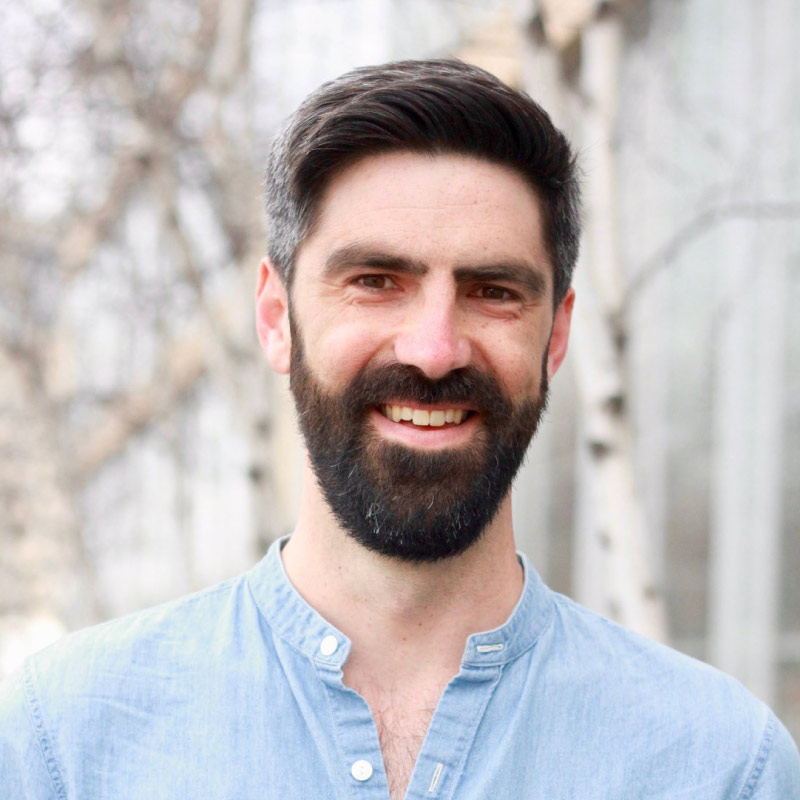
Matt Swanson
Director of Product DesignThe point is, there’s so much more to an individual than what data will tell you—it’s easy to have bias when you don’t have the behavioral side of the equation to make the best possible decision to engage someone. Plus, if you think you know someone based off of data, you may be engaging the right person, but with the wrong information, causing member abrasion and missing your opportunity to influence behavior.
Data does its job best when it’s blended with behavioral research—taking both into account equally. The powerful combination of the two is the recipe for super-powered personalization and an excellent way to combat bias.
3—Don’t Forget the Outliers
Outliers are the ones that fall outside of the average, causing their data to be thrown out so they aren’t taken into account, or they aren’t represented at all. It’s like when you decide to try out one of those personal styling services, like StitchFix. You fill out a long quiz about fit and color preferences and when you get to the end and are presented with a bunch of options that just don’t make sense. It’s frustrating—you spent an embarrassing amount of time filling out a profile stating repeatedly that you don’t like pattern or neon colors and yet the items shipped to you are floral dresses and brightly colored blouses? That’s an outlier.
Our data scientists wouldn’t recommend blindly trusting data—context is key. They’d suggest looking for the “why” behind it and considering any additional behavioral information you have that may contradict the output. However, with retail models like StitchFix, when they miss the mark with an outlier it’s generally okay because they don’t need to get everyone’s business. But when it’s healthcare, it’s important to engage everyone appropriately—especially the outliers because they are often the ones who need healthcare services the most.
To further complicate this, there are a lot of people out there that are hard to get to—many layers of barriers that can explain why a person becomes an outlier. Understanding variables that create outliers is critical to capture the why behind certain predictions.
In the end, we’re always trying to improve, build unbiased data models, and reach the people that need us the most. Constantly conducting test and control to see what’s working and what isn’t, coupled with seeing new populations emerge allow us to continue to evolve, to figure out what to do next, and how to more effectively engage with people that we may not have seen before asking “why”.